by Lei Fang and Xue Zhou
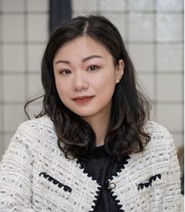
This blog is based on our recent publication: Zhou, X, Fang, L, & Rajaram, K (2025) ‘Exploring the digital divide among students of diverse demographic backgrounds: a survey of UK undergraduates’ Journal of Applied Learning and Teaching, 8(1).
Introduction – the widening digital divide
Our recent study (Zhou et al, 2025) surveyed 595 undergraduate students across the UK to examine the evolving digital divide across all forms of digital technologies. Although higher education is expected to narrow this divide and build students’ digital confidence, our findings revealed the opposite. We found that the gap in digital confidence and skills between widening participation (WP) and non-WP students widened progressively throughout the undergraduate journey. While students reported peak confidence in Year 2, this was followed by a notable decline in Year 3, when the digital divide became most pronounced. This drop coincides with a critical period when students begin applying their digital skills in real-world contexts, such as job applications and final-year projects.
Based on our study (Zhou et al, 2025), while universities offer a wide range of support such as laptop loans, free access to remote systems, extracurricular digital skills training, and targeted funding to WP students, WP students often do not make use of these resources. The core issue lies not in the absence of support, but in its uptake. WP students are often excluded from the peer networks and digital communities where emerging technologies are introduced, shared, and discussed. From a Connectivist perspective (Siemens, 2005), this lack of connection to digital, social, and institutional networks limits their awareness, confidence, and ability to engage meaningfully with available digital tools.
Building on these findings, this blog asks a timely question: as Generative Artificial Intelligence (GenAI) becomes embedded in higher education, will it help bridge this divide or deepen it further?
GenAI may widen the digital divide — without proper strategies
While the digital divide in higher education is already well-documented in relation to general technologies, the emergence of GenAI introduces new risks that may further widen this gap (Cachat-Rosset & Klarsfeld, 2023). This matters because students who are GenAI-literate often experience better academic performance (Sun & Zhou, 2024), making the divide not just about access but also about academic outcomes.
Unlike traditional digital tools, GenAI often demands more advanced infrastructure — including powerful devices, high-speed internet, and in many cases, paid subscriptions to unlock full functionality. WP students, who already face barriers to accessing basic digital infrastructure, are likely to be disproportionately excluded. This divide is not only student-level but also institutional. A few well-funded universities are able to subscribe to GenAI platforms such as ChatGPT, invest in specialised GenAI tools, and secure campus-wide licenses. In contrast, many institutions, particularly those under financial pressure, cannot afford such investments. These disparities risk creating a new cross-sector digital divide, where students’ access to emerging technologies depends not only on their background, but also on the resources of the university they attend.
In addition, the adoption of GenAI currently occurs primarily through informal channels via peers, online communities, or individual experimentation rather than structured teaching (Shailendra et al, 2024). WP students, who may lack access to these digital and social learning networks (Krstić et al, 2021), are therefore less likely to become aware of new GenAI tools, let alone develop the confidence and skills to use them effectively. Even when they do engage with GenAI, students may experience uncertainty, confusion, or fear about using it appropriately especially in the absence of clear guidance around academic integrity, ethical use, or institutional policy. This ambiguity can lead to increased anxiety and stress, contributing to wider concerns around mental health in GenAI learning environments.
Another concern is the risk of impersonal learning environments (Berei & Pusztai, 2022). When GenAI are implemented without inclusive design, the experience can feel detached and isolating, particularly for WP students, who often already feel marginalised. While GenAI tools may streamline administrative and learning processes, they can also weaken the sense of connection and belonging that is essential for student engagement and success.
GenAI can narrow the divide — with the right strategies
Although WP students are often excluded from digital networks, which Connectivism highlights as essential for learning (Goldie, 2016), GenAI, if used thoughtfully, can help reconnect them by offering personalised support, reducing geographic barriers, and expanding access to educational resources.
To achieve this, we propose five key strategies:
- Invest in infrastructure and access: Universities must ensure that all students have the tools to participate in the AI-enabled classroom including access to devices, core software, and free versions of widely used GenAI platforms. While there is a growing variety of GenAI tools on the market, institutions facing financial pressures must prioritise tools that are both widely used and demonstrably effective. The goal is not to adopt everything, but to ensure that all students have equitable access to the essentials.
- Rethink training with inclusion in mind: GenAI literacy training must go beyond traditional models. It should reflect Equality, Diversity and Inclusion principles recognising the different starting points students bring and offering flexible, practical formats. Micro-credentials on platforms like LinkedIn Learning or university-branded short courses can provide just-in-time, accessible learning opportunities. These resources are available anytime and from anywhere, enabling students who were previously excluded such as those in rural or under-resourced areas to access learning on their own terms.
- Build digital communities and peer networks: Social connection is a key enabler of learning (Siemens, 2005). Institutions should foster GenAI learning communities where students can exchange ideas, offer peer support, and normalise experimentation. Mental readiness is just as important as technical skill and being part of a supportive network can reduce anxiety and stigma around GenAI use.
- Design inclusive GenAI policies and ensure ongoing evaluation: Institutions must establish clear, inclusive policies around GenAI use that balance innovation with ethics (Schofield & Zhang, 2024). These policies should be communicated transparently and reviewed regularly, informed by diverse student feedback and ongoing evaluation of impact.
- Adopt a human-centred approach to GenAI integration: Following UNESCO’s human-centred approach to AI in education (UNESCO, 2024; 2025), GenAI should be used to enhance, not replace the human elements of teaching and learning. While GenAI can support personalisation and reduce administrative burdens, the presence of academic and pastoral staff remains essential. By freeing staff from routine tasks, GenAI can enable them to focus more fully on this high-impact, relational work, such as mentoring, guidance, and personalised support that WP students often benefit from most.
Conclusion
Generative AI alone will not determine the future of equity in higher education, our actions will. Without intentional, inclusive strategies, GenAI risks amplifying existing digital inequalities, further disadvantaging WP students. However, by proactively addressing access barriers, delivering inclusive and flexible training, building supportive digital communities, embedding ethical policies, and preserving meaningful human interaction, GenAI can become a powerful tool for inclusion. The digital divide doesn’t close itself; institutions must embed equity into every stage of GenAI adoption. The time to act is not once systems are already in place, it is now.
Dr Lei Fang is a Senior Lecturer in Digital Transformation at Queen Mary University of London. Her research interests include AI literacy, digital technology adoption, the application of AI in higher education, and risk management. [email protected]
Professor Xue Zhou is a Professor in AI in Business Education at the University of Leicester. Her research interests fall in the areas of digital literacy, digital technology adoption, cross-cultural adjustment and online professionalism. [email protected]